With the start of the school year approaching, educators are wondering how artificial intelligence, or AI, may be used in learning environments, including by students for writing papers.
Bill Hart-Davidson has been researching AI since he first came to Michigan State University in 2004 and launched a research center about writing in digital environments. Hart-Davidson is Associate Dean of Research in the College of Arts & Letters and a Professor of Writing, Rhetoric, and Cultures. He also co-invented Eli Review, an online writing and peer feedback tool for students.
Hart-Davidson discusses OpenAI’s product ChatGPT and how generative AI is starting to change the way we write while also making review and revision increasingly important. He also explores some of the challenges with AI models related to language, bias, and transparency.
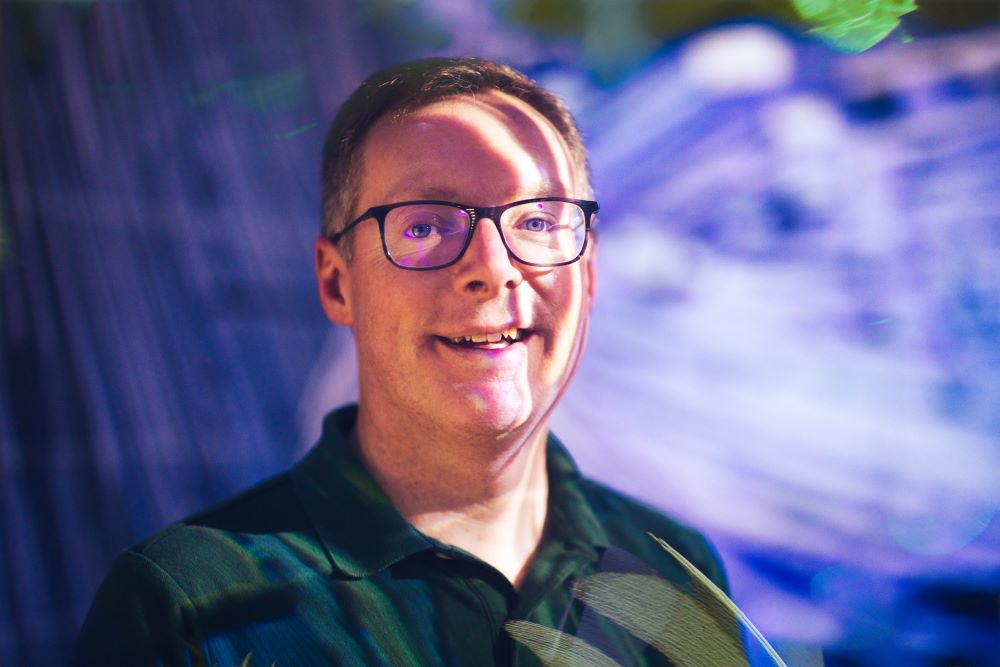
How is AI affecting faculty in higher education?
The theme of academic integrity keeps coming up, and that’s not necessarily plagiarism or violations of academic integrity, but a question about whether we can trust what we’re seeing from students as their own work. There is also just that initial shock from faculty thinking, “Am I going to have to redo my whole class now?” For faculty, that feels overwhelming, especially at the pace that we are seeing AI change.
There is a sense of wonder, excitement, and amazement at what generative AI can do now and what it might be capable of in the future. People also are realizing that this is going to change a lot of things in a hurry. AI and creativity are a source of equal amounts of wonder and fear right now.
How might AI create a new awareness of writing as a part of learning?
Writing is a wonderful mode of learning even without creating a text that you would show to anyone else. We use writing to organize our own thinking, to take a series of complex ideas, and to feel like we have more confidence and ownership over the shape of them. That aspect of what writing does for us is likely to never lose its value in an educational setting.
A simplified version of the writing process that my colleagues and I teach students is write, review, revise, and then repeat. It’s how we become better writers, which is what we’re often more concerned with in a writing course — helping the writers, not just the writing, improve! Of course, this is also how you get to a better draft.
Generative AI gives us a way to do that first step — draft — much faster, so we can get to a pretty good draft quickly. We will still need review and revision in almost every case in which we want to build trust that the writing act has some integrity. Review and revision are the durable human skills of writing. Arguably, we now need an even better, more nuanced, and more diverse range of review and revision skills.
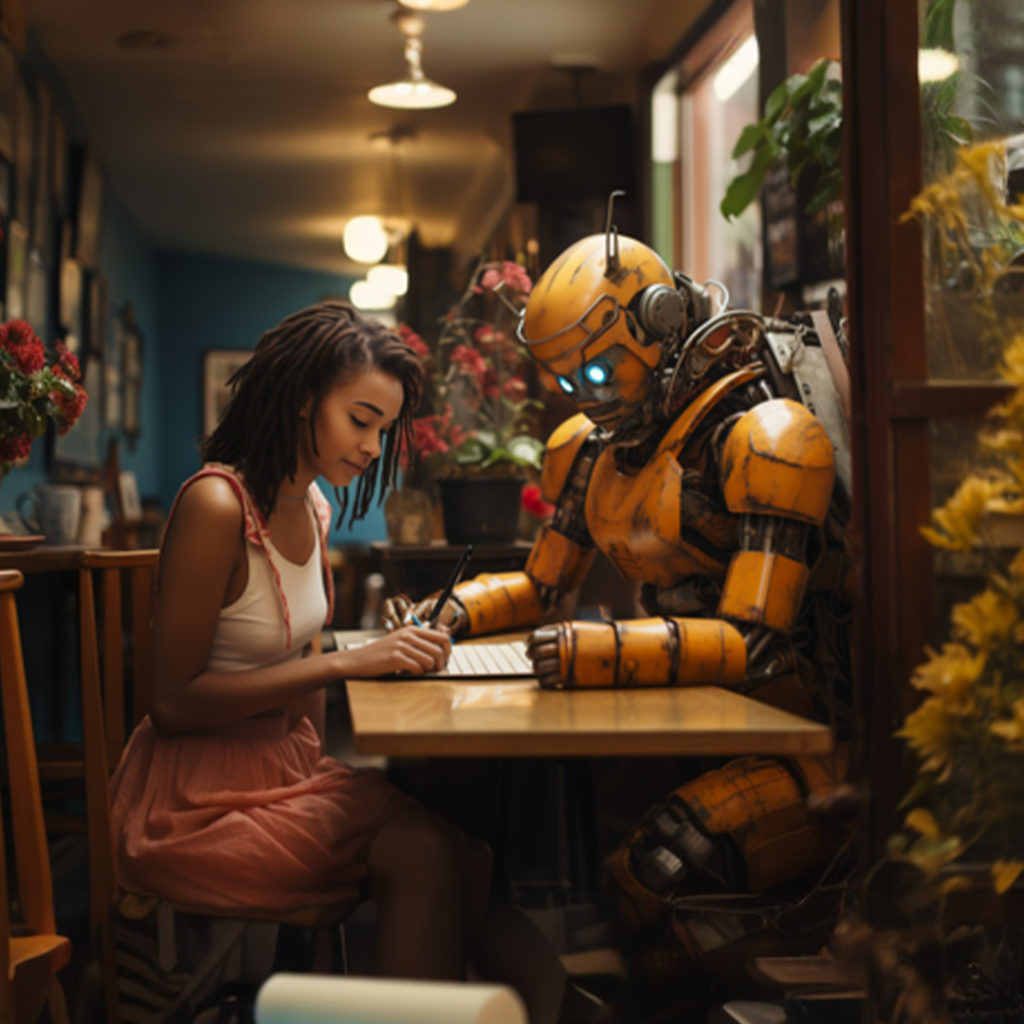
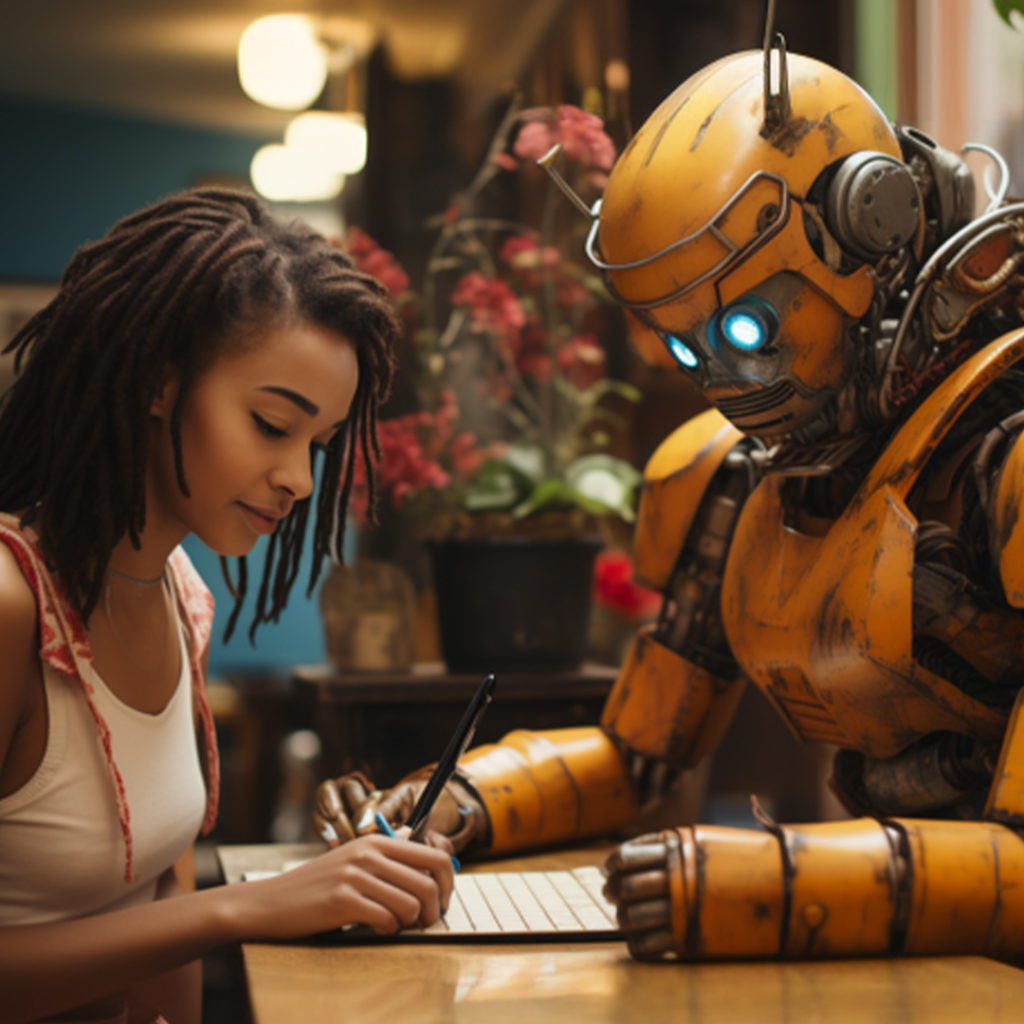
How do AI tools like ChatGPT fall short in text output?
ChatGPT is designed to produce the most common or most reasonable way to say something in response to a prompt. So what it delivers will always be something more conventional.
This is why we need to help students learn to become better and more nuanced readers, responders, and revisers. If it’s super easy to create the conventional, we’ll quickly develop an economy around interesting text that values anything but the conventional because that’s so cheap and easy.
ChatGPT as a language model has no truth filter. There’s no way for it to know what’s accurate when it produces something versus what’s inaccurate. People are trying to solve that with other layers of deep-learning training. In the meantime — and in any case where the stakes are high — we’re going to want humans to do an accuracy review. That’s a piece of behavior in the act of writing that engenders trust, and we can’t give that away or else we just won’t trust the results as much.
How can AI complement learning?
I would encourage people to experiment with using AI whenever they feel they’re in a learning situation for a new type of writing — perhaps trying to write in a genre that’s new to you. Use AI to provide an example. Philosophers like Socrates and Cicero encouraged people to learn by imitation, by looking at popular examples of a thing, analyzing them, and then doing something similar.
You’re not exactly copying the same words in the exact order when you imitate. What you should be doing is looking for repeatable moves, building a repertoire. When you are confronted with a common situation, you know what pattern to follow. You don’t need to know the exact words and the exact order, but you know the moves to make.
In that context, I think the ability to conjure up a pretty good example of just about anything is a powerful tool. It’s an accelerator to learning.
How could AI affect language more broadly?
Language conventions and language standards have material consequences for people. In the past in this country and in other countries, the ways you write and talk have been consequential for people in both negative and positive ways. They have been used to oppress people.
We use a term “standard written English” and, largely, we view it as a good thing, but standard written English is by its definition the middle part of that distribution. A lot of the specific cultural meanings and the way people talk in their homes and their communities is not represented there. That has a racial discrimination history in the U.S. It has a nationality and language linguistic community distinction. It has gender implications.
We’re in a situation now with the pace of AI where we might be moving rapidly toward a new kind of standard English — one that isn’t determined by people or isn’t really reinforced by rules or editors, but that is simply reproduced at a shockingly fast rate by a computer. It has the potential to erase linguistic differences and, if not erase them, make them seem less valid. I think that’s a legitimate worry.
It’s not about making a prior judgment related to whose language matters more, but rather having a nuanced understanding around how linguistic communities develop, how they change over time, and how they convey more than just straightforward semantic meaning in our language choices. They also carry culture and tradition. We don’t want to get rid of all those things.
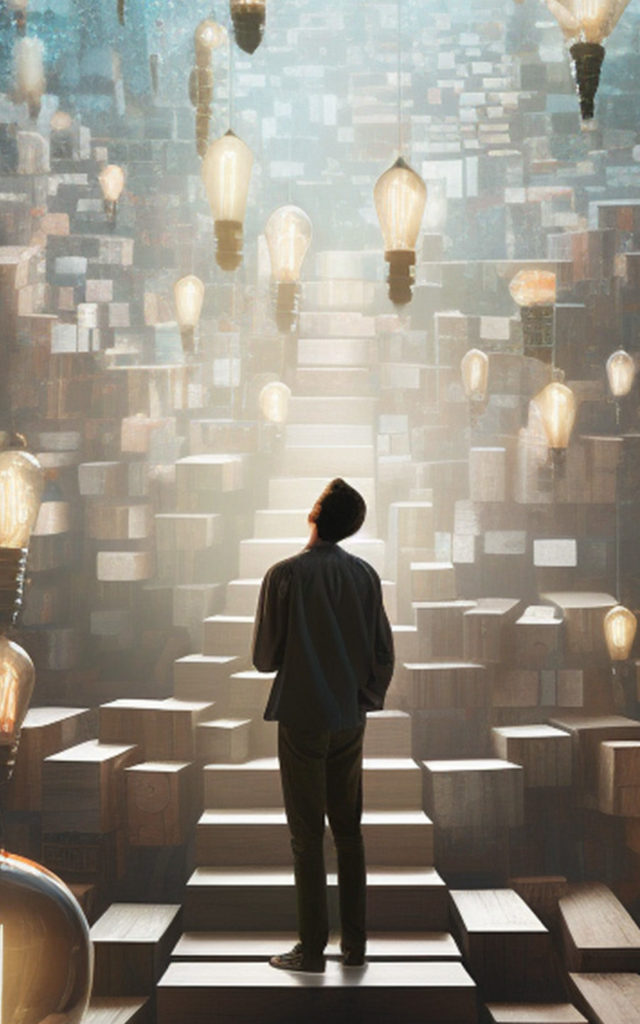
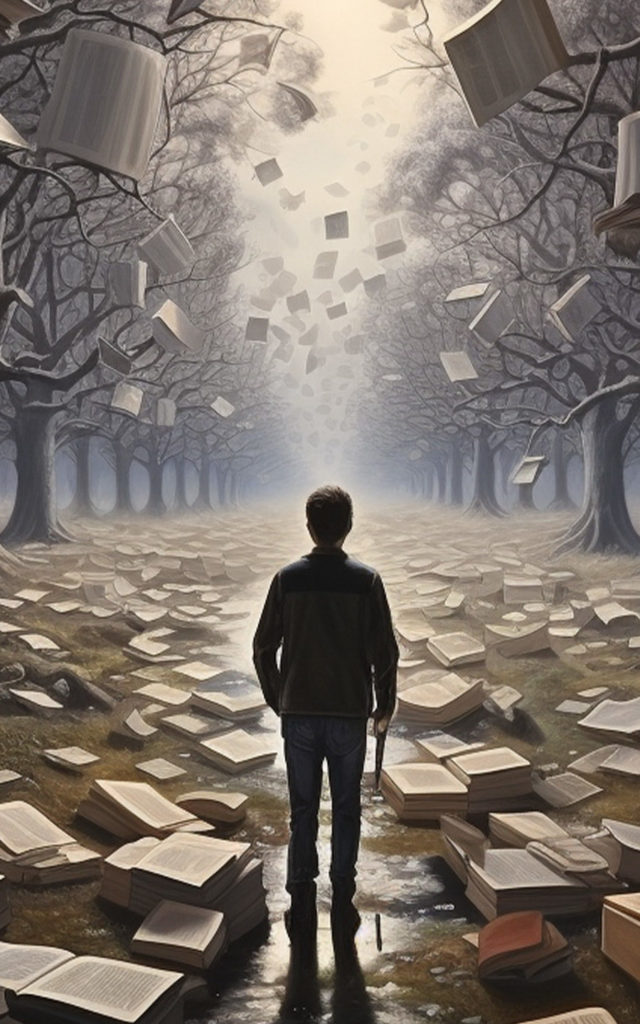
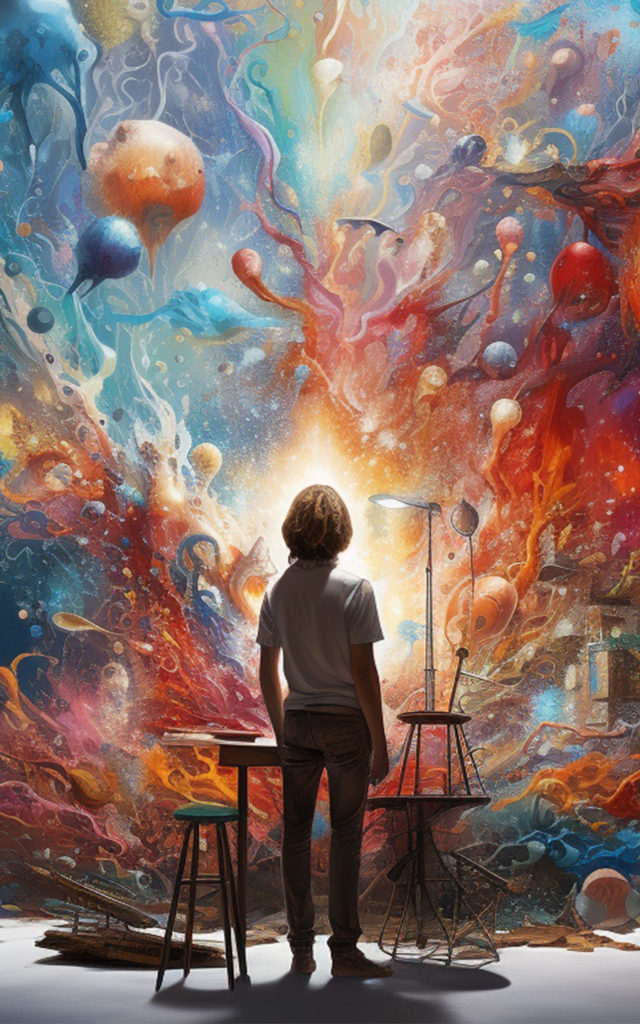
Where do you think some of the fears related to AI stem from?
We’ve been asked to accept AI technologies without a lot of disclosure about the text on which they’ve been trained. We were never part of a process, even if our texts are represented in those training materials and even if they show up in the tools and spaces that we now inhabit. We haven’t been asked for our consent, so we haven’t had a chance to opt out. We haven’t had a chance to push back. We haven’t had a chance to correct. We might still have some of those chances, but this is a wildly unregulated technology right now.
We know very little about how machine language learning models work, and we also don’t have any way to regulate models or ask the makers of these technologies to adhere to a particular standard for checking for bias. I think that’s coming next.
If people are really going to start to use AI to carry out high-stakes operations, then we’re going to need ways to detect and correct for these biases, even if just as a matter of making AI tools commercially viable. Then we need to address all these other forms of injustice that can not only exist on the surface, but also can be perpetuated with the way we use language. All the -isms — racism and sexism for example — often start off as linguistic habits that we carry over into other kinds of actions. If they’re present in the language and the AI training set of data, they’re going to be present in the output of the AI model.
Media Contacts: Beth Bonsall, Alex Tekip